
GEO:AI
The scientific field of geospatial artificial intelligence (GEO:AI) was recently formed from combining innovations in spatial science with the rapid growth of methods in artificial intelligence (AI), particularly machine learning (e.g., deep learning), data mining, and high-performance computing to glean meaningful information from spatial big data.
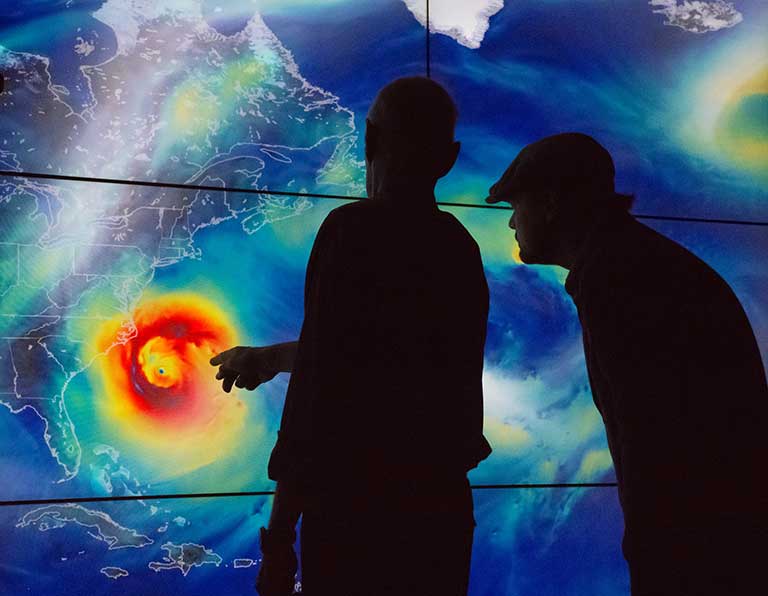
GEO:AI is highly interdisciplinary, bridging many scientific fields including geography, computer science, engineering, statistics, and spatial science. Here In UF Geography, we have a long history of faculty engaged In using AI and machine-learning approaches to solve geographical and societal problems. Through our GEO:AI research projects, certificate and courses, we are able to link our existing expertise in medical geography and global health, GIScience, extreme events and hazards, earth systems science, sustainability and global change as well as with our expanded expertise in AI and atmospheric sciences, to address real world problems and to train a workforce in this exciting new field.
GEO:AI Faculty & Research
Our areas of expertise range from the geospatial modeling of disease and genetic features, modelling complex social-ecological problems, understanding atmospheric systems, environmental remote sensing, coastal analysis and extreme events, and more. Dr. Jane Southworth, the Geography Department Chair, serves on the “CLAS AI Curriculum”, the “UF AI Curriculum Committee” and “UF AI Research Committee”, and is Co-Chair of the AI Across the Curriculum initiative for the next QEP.
Examples of Faculty Projects linked to AI
Geospatial Modeling of Disease and Genetic Features
- Dr. Michael Norris and Dr. Jason Blackburn employ spatial modeling and geographic information systems, remote sensing, and micro- and molecular biology.
- Dr. Sadie Ryan uses lab modeling approaches such as SDMs, machine learning to detect arbovirus cases from patient records, and exploratory hierarchical RF modeling, ongoing examples of using AI/machine learning in research.
Complex Social-Ecological Problem Models
- Dr. Michael Binford has a current NSF-funded project which uses machine-learning AI approaches to analyze satellite remote sensing and other geospatial data for categorizing land-use at the continental scale, with plans to incorporate land management into Earth Systems Models.
- Dr. Jane Southworth uses machine-learning AI approaches to analyze satellite remote sensing and other geospatial data big data, in currently funded NSF CNH Proposal on large scale land transactions in Ethiopia.
- Dr. Robert T. Walker’s work integrates remote sensing, spatial statistics, and ethnographic field data into studies of land cover change processes.
Atmospheric Systems, Environmental Remote Sensing, and Coastal Analysis and Extreme Events
- Dr. Kevin Ash’s primary analysis tools are geospatial and statistical modeling in the context of weather and climate hazards.
- Dr. Johanna Engström’s focus areas are Sustainability, Climatology, Climate Change, Artificial Intelligence, Applied Machine Learning, and GIScience.
- Dr. David Keellings’ focus areas are Climatology, Climate Change, Artificial Intelligence, Applied Machine Learning, Extreme Events, and Hazards.
- Dr. Corene Matyas has a current NSF-funded project analyzing data from ground-based weather radars and radar from satellites (big spatial data) to examine hurricane rainband spatial patterns and relationship with environmental moisture.
- Dr. Stephen Mullens has an NSF AGS proposal for using machine learning to evaluate climate change effects on winter storms
- Dr. Katy Serafin has a current U.S. Coastal Research Program funded project that uses multivariate statistical models, hydrodynamic numerical models, and machine learning algorithms to develop an efficient and scalable hybrid modeling framework for quantifying graduated compound flood risk along coastal river systems.
- Dr. Berry Wen’s focus areas are the analysis of massive remote sensing data from NASA missions and NOAA weather radar programs aided by machine learning technologies, so as to better understand the Earth’s climate systems and to monitor and forecast water-related natural hazards, such as hurricanes, tornadoes, and flash floods.
Spatiotemporal Dynamics
- Dr. Yujie Hu is using machine learning to analyze spatiotemporal dynamics of criminal behaviors during crises to assist crime prevention.
2022 AI Hires
GEO:AI Certificate — The Integration of Geography and AI
GEO:AI provides novel approaches for addressing a variety of geospatial challenges in the real world. This certificate develops transferable skills in GEO:AI to address issues impacting our environment and societies. GEO:AI training is critical given projected growth of ~16% in this industry by 2050.
Learn more about the GEO:AI Certificate
Courses in AI
Integration of Geography and AI, or GeoAI (a subfield of spatial data science), provides novel approaches for addressing a variety of geospatial problems in the natural environment and our human society. Hands-on computing labs using real-world geospatial data to address such AI topics as: image classification, object detection, scene segmentation, simulation and interpolation, retrieval and question answering, on-the-fly data integration, and geo-enrichment.
Credit Hours: 3 | Prerequisites: Any 3000 level or higher GIS prefix course or permission of instructor.
An introduction to geodata analysis using R. The course will be taught from the perspective of geographical and climate data analysis but serves as a broad introduction to the high-level programming language, R, as well as applied spatial data analysis. Students will load and manipulate data of different types, perform a variety of statistical analyses, generate graphical output, and create productive workflows using R alone. The primary outcome will be to facilitate students’ use of R to analyze data of their own choosing on a final project. Students will present these methods to the class for others to critique, analyze and learn from. Code sharing and re-use is highly emphasized, as is collaboration. The course is designed as a 1-hour lecture plus 2-hour lab each week.
Credit Hours: 3 | Prerequisites: Any 3000 level or higher GIS prefix course or permission of instructor.
It is often the case that real-world systems can be represented as networks of many interacting components. Examples include information (the World Wide Web, citation), social (friendship, classmates), physical (transportation, distribution), biological (food chain, brain) systems, and so forth. Many of these networks are essentially spatial, meaning each node can be associated with a location somewhere in the real world. Based on recent advances in network science and GIScience, this course teaches the fundamental concepts, models, and techniques for describing, visualizing, measuring, and analyzing networks. It also introduces their applications in geography, transportation, social science, etc. A series of labs using the popular network analysis package Gephi and GIS software ArcGIS are also designed to help students gain hands-on experience in visualizing and analyzing networks.
Credit Hours: 3 | Prerequisites: Entry level knowledge of statistics or instructor permission. Prior experience with ArcGIS is preferred.
Focuses on how the Earth’s surface is visualized, explored, and analyzed in digital formats (e.g. maps, satellite images, aerial photos). Provides an introduction to fundamental concepts of digital geographic data to understand the Earth environment and human society based on the vast quantities of geographic information in our ever-changing world.
Credit Hours: 3 | Prerequisites: None
Data drives today’s world and over 80% of that data is geographic. Increasing volume and varying formats of these geospatial big datasets have posed new challenges. Introduces relevant concepts (e.g., 5 V’s) and techniques (e.g., cloud computing) of big (spatial) data as well as its applications in the real world. Hands-on experiences with Volunteered Geographic Information (VGI), analyzing geotagged tweets, and visualizing spatial datasets.
Credit Hours: 3 | Prerequisites: None
Geographic Information Systems (GIS) as the technology for creation, modification, display and analysis of spatial information. Develops knowledge of GIS, competence in geographic databases and familiarity with computer software and hardware.
Credit Hours: 4 | Prerequisites: GEO 2200, GEO 3162C and 2000-level human geography course.
Focuses on the design of GIS-based models to address health and healthcare issues. Topics include a conceptual framework, landscape epidemiology models, disease diffusion models, health accessibility, human health behavior and location-allocation of health services. Laboratory section provides hands-on experience applying these models with GIS tools.
Credit Hours: 3 | Prerequisites: GIS 3043 and (STA 2023 or GEO 3162C).
Introduces the theory and application of digital imagery data in geographical research with a hands-on, lab-based approach.
Credit Hours: 4 | Prerequisites: Junior or Senior Standing.
Introduces basic programming concepts; instruction in popular programming languages for geospatial processing, applications, and modeling in ArcGIS environment.
Credit Hours: 3 | Prerequisites: GIS 3043C or equivalent.
Many phenomena of interest in physical, social and cyber environments can be thought of as networks within geographic context. Teaches methods for analyzing these spatial networks, and introduces their applications in geography, transportation, hydrology, epidemiology, social science, etc.
Credit Hours: 3 | Prerequisites: Entry level knowledge of statistics or instructor permission. Prior experience with ArcGIS is preferred.
Introduces fundamentals and practices of advanced geostatistical analysis (kriging), which addresses optimal spatial interpolation. Geostatistics are currently applied in diverse disciplines such as geography, geology, engineering, hydrology, urban studies and epidemiology.
Credit Hours: 3 | Prerequisites: (STA 2023 or GEO 3162C or equivalent) and GIS 3043 or equivalent or instructor permission.
Integration of Geography and AI, or GeoAI (a subfield of spatial data science), provides novel approaches for addressing a variety of geospatial problems in the natural environment and our human society. Hands-on computing labs using real-world geospatial data to address such AI topics as: image classification, object detection, scene segmentation, simulation and interpolation, retrieval and question answering, on-the-fly data integration, and geo-enrichment.
Credit Hours: 3 | Prerequisites: Any 3000 level or higher GIS prefix course [GIS3XXX, GIS4XXX] or permission of instructor.
Introduction to geodata analysis using programming. Broad introduction to the programming language as well as applied spatial data analysis. Facilitates students’ use of programming to analyze data of their own choosing on a final project. Code sharing and re-use is highly emphasized, as is collaboration.
Credit Hours: 3 | Prerequisites: Any 3000 level or higher GIS prefix course or permission of instructor.
Geographic and cartographic techniques for geospatial analysis of risk, vulnerability, and resilience using ArcGIS. Learn to utilize physical and human geographic datasets for multiple hazard contexts including hydrometeorological, climatological, and geophysical hazards.
Credit Hours: 3 | Prerequisites: GIS 3043 or URP 4273 with minimum grade of C.
Focuses on GIS applications in spatial analysis and ecology to address common research issues related to zoonotic diseases which affect animals and humans.
Credit Hours: 3 | Prerequisites: GIS 3043 or GIS 3420C, or equivalent.
Instruction on geographic and cartographic techniques for geospatial analysis of population, demographic, and socioeconomic data using ArcGIS Pro. Students identify and utilize current and historical secondary population data sources for GIS analysis of population changes, and for mapping of segregation, inequality, and well-being indicators.
Credit Hours: 3 | Prerequisites: GIS 3043 or GIS 3001C or URP 4273 with minimum grades of C, or GEO 3430 or SYD 4020.
Provides a fundamental understanding of the Python programming language with a core focus on ingesting, displaying, and analyzing observational meteorological data and numerical weather model data.
Credit Hours: 3 | Prerequisites: MET 1010 or GEO 2242; Corequisite: MET 3573.
Overview of radar and satellite remote sensing as used in the atmospheric sciences, including the principles of atmospheric radiative transfer, the retrieval of atmospheric variables, and emphasis on geospatial interpretation of imagery for different weather systems.
Credit Hours: 3 | Prerequisites: PHY 2049 and MET 3503.
Meteorological and climatological concepts related to hurricanes. Forecasting current activity, researching past storms and analyzing storm structure, damage and future trends.
Credit Hours: 3 | Prerequisites: MET 3503 or GEO 3250.
Atmospheric teleconnections are recurring large-scale patterns of pressure and circulation anomalies. They can influence temperature, rainfall, storm tracks and jet stream location and intensity. Examines how these patterns were discovered, how the index that characterizes the phase of each teleconnection is calculated and the weather associated with different phases.
Credit Hours: 3 | Prerequisites: MET 3503 or GEO 3250 with a minimum B- grade.
How atmospheric data are collected and analyzed for meteorologic and climatologic-scale research. Where various types of data are obtained and how to analyze data to answer specific research questions.
Credit Hours: 3 | Prerequisites: GEO 3250 or MET 3503 or MET 4532
Introduces regression models capable of dealing with spatial auto-correlation; develop statistical models and estimate with computer software.
Credit Hours: 3 | Prerequisites: GEO 4167C or equivalent.
Investigates techniques for evaluating the risks of extreme events related to water in our environment. Presents data and methodologies for estimating the rarity of phenomena including excessive rainfall totals, high and low river levels, coastal storm surge and waves, and drought.
Credit Hours: 3 | Prerequisites: GEO 3162C or STA 3032 or permission of instructor. | Attributes: Satisfies 6000 Words of Writing Requirement.
Introduces the spatial epidemiology of vector-borne diseases (VBDs) and geospatial methods for monitoring, mapping and modeling them. Provides hands-on experiences for mapping and modeling risk of VBDs via GIS-based labs.
Credit Hours: 3 | Prerequisites: GEO 3452 or GIS 3043 or permission of the instructor.