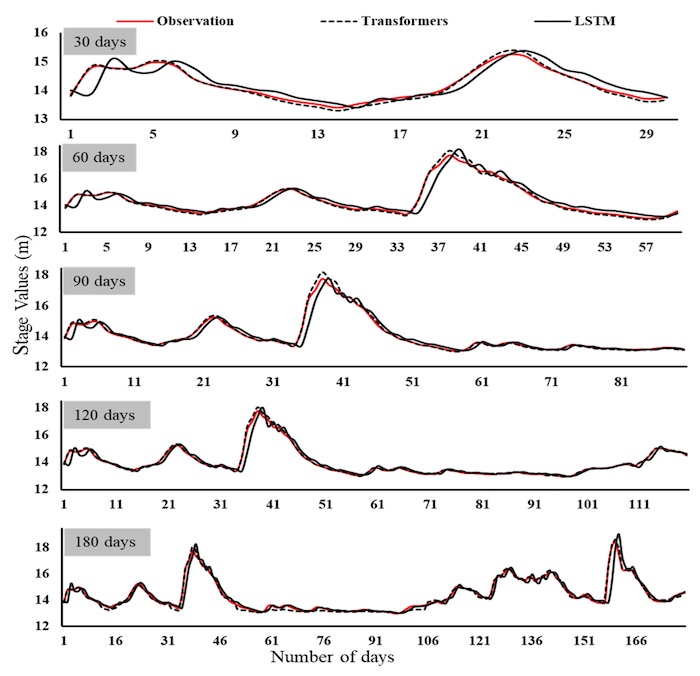
AMANAMBU, MOSSA, CHEN – Hydrological Drought Forecasting Using a Deep Transformer Model
Amobichukwu Amanambu, Joann Mossa, Yin-Hsuen Chen
Article first published online: 9 November 2022
DOI: https://doi.org/10.3390/w14223611
ABSTRACT: Hydrological drought forecasting is essential for effective water resource management planning. Innovations in computer science and artificial intelligence (AI) have been incorporated into Earth science research domains to improve predictive performance for water resource planning and disaster management. Forecasting of future hydrological drought can assist with mitigation strategies for various stakeholders. This study uses the transformer deep learning model to forecast hydrological drought, with a benchmark comparison with the long short-term memory (LSTM) model. These models were applied to the Apalachicola River, Florida, with two gauging stations located at Chattahoochee and Blountstown. Daily stage-height data from the period 1928–2022 were collected from these two stations. The two deep learning models were used to predict stage data for five different time steps: 30, 60, 90, 120, and 180 days. A drought series was created from the forecasted values using a monthly fixed threshold of the 75th percentile (75Q). The transformer model outperformed the LSTM model for all of the timescales at both locations when considering the following averages: MSE=0.11, MAE=0.21, RSME=0.31, and R^2=0.92 for the Chatahoochee station, and MSE=0.06, MAE=0.19, RSME=0.23, and R^2=0.93 for the Blountstown station. The transformer model exhibited greater accuracy in generating the same drought series as the observed data after applying the 75Q threshold, with few exceptions. Considering the evaluation criteria, the transformer deep learning model accurately forecasts hydrological drought in the Apalachicola River, which could be helpful for drought planning and mitigation in this area of contested water resources, and likely has broad applicability elsewhere.
Read the full publication in Water.