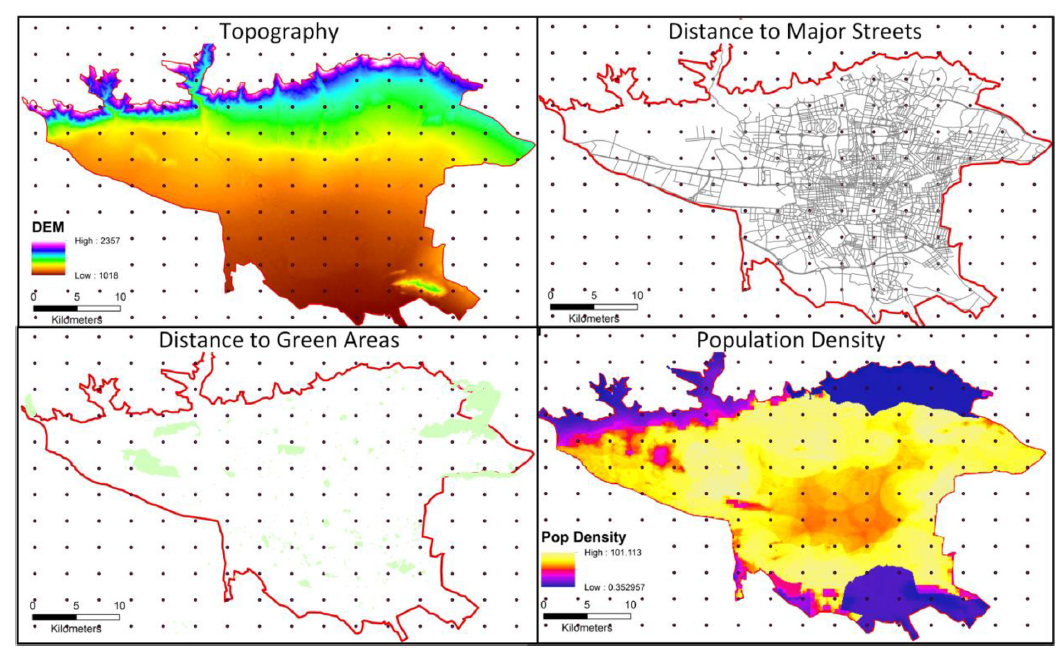
Rezaali, M., Jahangir, M. S., Fouladi-Fard, R., & Keellings, D. (2024). An ensemble deep learning approach to spatiotemporal tropospheric ozone forecasting: A case study of Tehran, Iran. Urban Climate, 55, 101950. DOI: https://doi.org/10.1016/j.uclim.2024.101950.
This study proposes a novel framework for spatiotemporal forecasting of Ground-level Ozone Concentration (GOC) using advanced machine learning techniques, including Artificial Neural Networks (ANN), Regularized Extreme Learning Machines (RELM), Long Short-Term Memory (LSTM), Bidirectional LSTM (BiLSTM), Gated Recurrent Unit (GRU), and their wavelet counterparts. Data from eight air quality stations in Tehran, Iran, were employed for training and testing. The impact of wavelet decomposition on the reliability of machine learning models was evaluated using the Data Frame Coverage Index (DFCI). Among standalone Deep Learning (DL) models, LSTM exhibited the highest reliability with the lowest average DFCI of 0.65. Wavelet-based DL models (WLSTM and WGRU) showed similar performance with average DFCIs of 0.68 and 0.69, respectively. The wavelet and non-wavelet versions of Shallow Learning (SL) models performed comparably with average DFCIs near 0.7. Continuous Ranked Probability Score (CPP) analysis revealed the advantage of DL models in incorporating wavelet-decomposed data. SL models tended to underestimate predictive uncertainty, with slight improvements via wavelet decomposition. WBiLSTM outperformed all models in terms of probabilistic reliability indices, i.e., α and ξ with the values of 0.83 and 0.88, respectively. Overall, this study demonstrates the potential of wavelet-based DL models for accurate and reliable spatiotemporal forecasting of GOC. The proposed framework can be valuable for air quality management in regions with elevated GOC levels.