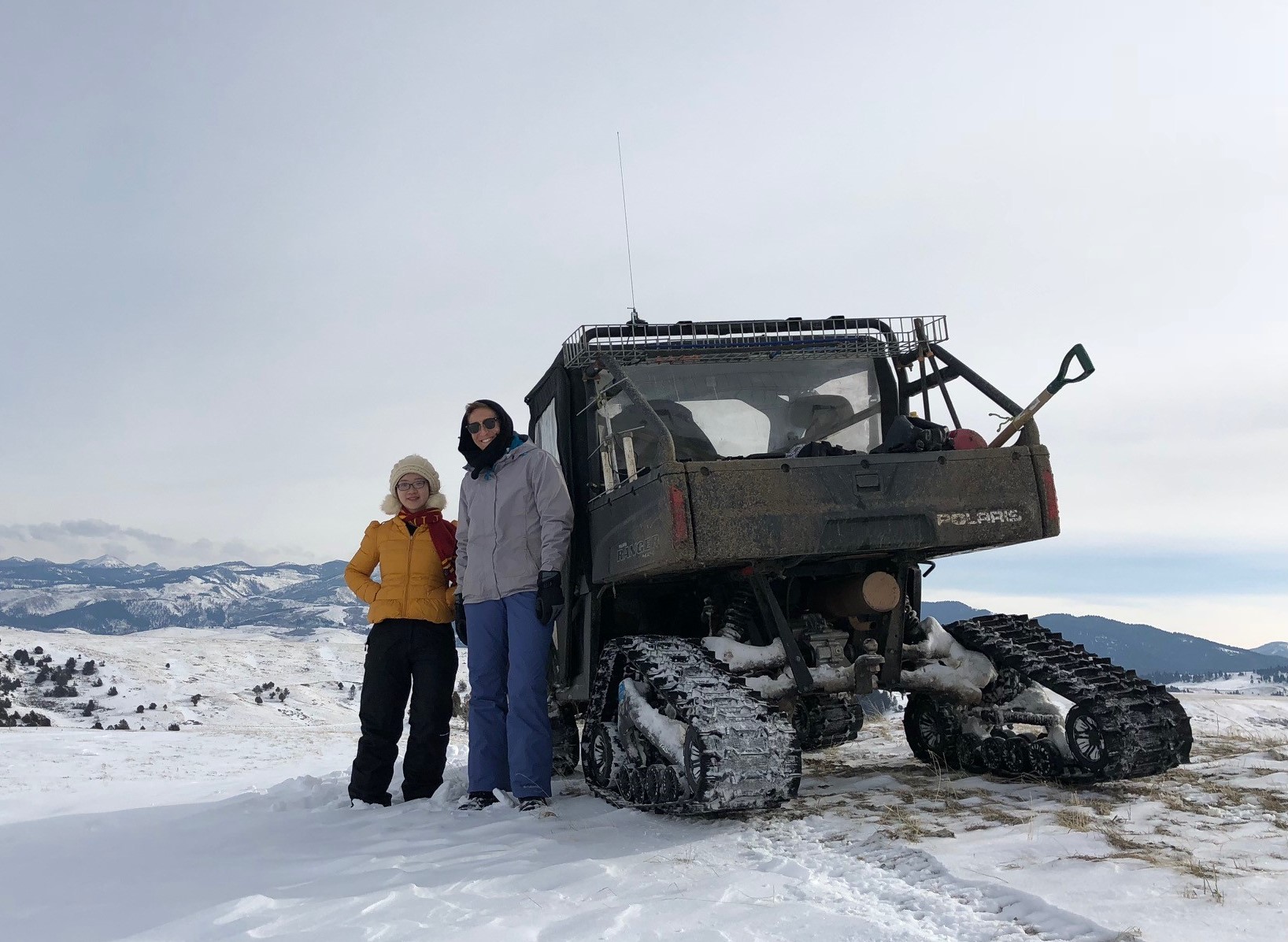
Ecological niche models (ENMs) are widely used to predict the geographic distribution of species and are applied across taxa. Within the geography department, ENMs have been used to map hosts, vectors, and pathogens for MedGeo studies, species of conservation concern, and invasive species potential. Such models rely on relating non-random patterns of occurrences, locations where species have been confirmed, to environmental variables, such as temperature, precipitation, and vegetation index. The selection of variables remains a critical step in the modeling process and one of the greatest challenges.
Researchers aim to build parsimonious models, those with the fewest environmental variables to produce the most accurate model. In the latest SEER Lab publication in PeerJ, MedGeo PhD Alum Dr, Anni Yang, MedGeo Postdoc Alum Dr. Juan Pablo Gomez, and MedGeo Associate Professor Dr, Jason Blackburn tackled this challenge through the development of a new variable selection tool and workflow for the genetic algorithm for rule-set prediction (GARP). GARP is a widely used machine learning approach to model species’ distributions. In this study, the team introduced the unimportance index (UI) for ranking variable contributions in GARP experiments using a set of R-based functions within the GARPTools R Package. SEER Lab developed the GARPTools package with Dr. Katie Haase, a UF PhD Alum now on faculty at Austin Peay State University. The version of GARP required for applying this approach can be found online https://github.com/jkblackburn/DesktopGARP1.1.3. The GARPTools pacakage is freely available online https://github.com/cghaase/GARPTools and the code from the paper can be found at https://github.com/jkblackburn/Trufum_GARPTools.
Read Exploring environmental coverages of species: a new variable contribution estimation methodology for rulesets from the genetic algorithm for rule-set prediction at PeerJ.