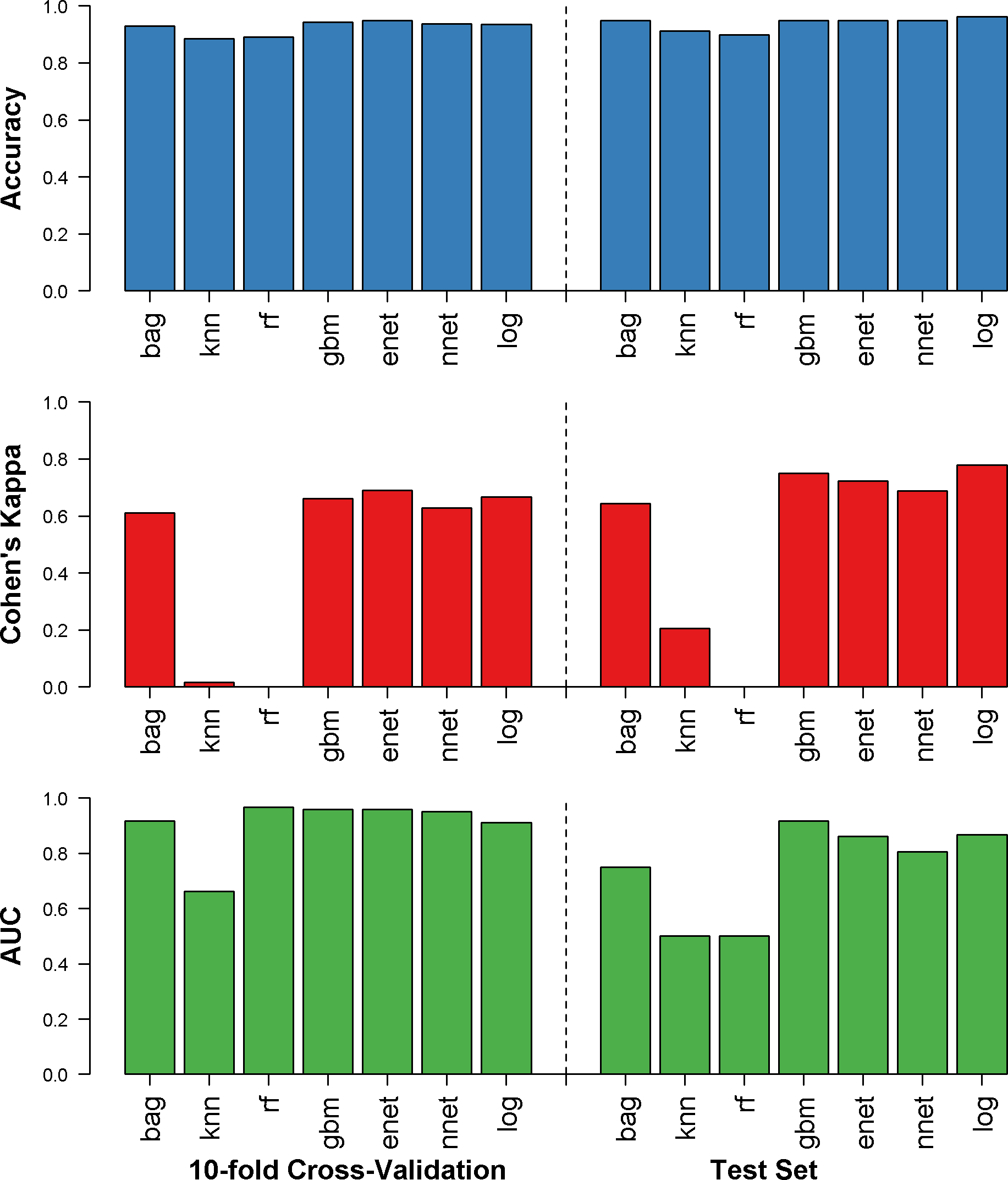
SIPPY, RYAN – Severity Index for Suspected Arbovirus (SISA): Machine learning for accurate prediction of hospitalization in subjects suspected of arboviral infection
Rachel Sippy, Daniel F. Farrell, Daniel A. Lichtenstein, Ryan Nightingale, Megan A. Harris, Joseph Toth, Paris Hantztidiamantis, Nicholas Usher, Cinthya Cueva Aponte, Julio Barzallo Aguilar, Anthony Puthumana, Christina D. Lupone, Timothy Endy, Sadie J. Ryan, and Anna M. Stewart Ibarra
Article first published online: 14 FEB 2020 PLoS Neglected Tropical Diseases
DOI: 10.1371/journal.pntd.0007969
ABSTRACT:
Background
Dengue, chikungunya, and Zika are arboviruses of major global health concern. Decisions regarding the clinical management of suspected arboviral infection are challenging in resource-limited settings, particularly when deciding on patient hospitalization. The objective of this study was to determine if hospitalization of individuals with suspected arboviral infections could be predicted using subject intake data.
Methodology/Principal findings
Two prediction models were developed using data from a surveillance study in Machala, a city in southern coastal Ecuador with a high burden of arboviral infections. Data were obtained from subjects who presented at sentinel medical centers with suspected arboviral infection (November 2013 to September 2017). The first prediction model—called the Severity Index for Suspected Arbovirus (SISA)—used only demographic and symptom data. The second prediction model—called the Severity Index for Suspected Arbovirus with Laboratory (SISAL)—incorporated laboratory data. These models were selected by comparing the prediction ability of seven machine learning algorithms; the area under the receiver operating characteristic curve from the prediction of a test dataset was used to select the final algorithm for each model. After eliminating those with missing data, the SISA dataset had 534 subjects, and the SISAL dataset had 98 subjects. For SISA, the best prediction algorithm was the generalized boosting model, with an AUC of 0.91. For SISAL, the best prediction algorithm was the elastic net with an AUC of 0.94. A sensitivity analysis revealed that SISA and SISAL are not directly comparable to one another.
Conclusions/Significance
Both SISA and SISAL were able to predict arbovirus hospitalization with a high degree of accuracy in our dataset. These algorithms will need to be tested and validated on new data from future patients. Machine learning is a powerful prediction tool and provides an excellent option for new management tools and clinical assessment of arboviral infection.
Author summary
Patient triage is a critical decision for clinicians. Patients with suspected arbovirus infection are difficult to diagnose as symptoms can be vague and molecular testing can be expensive or unavailable. Determining whether these patients should be hospitalized or not can be challenging, especially in resource-limited settings. Our study included data from 543 subjects with a diagnosis of suspected dengue, chikungunya, or Zika infection. Using a machine learning approach, we tested the ability of seven algorithms to predict hospitalization status based on the signs, symptoms, and laboratory data that would be available to a clinician at patient intake. Using only signs and symptoms, we were able to predict hospitalization with high accuracy (94%). Including laboratory data also resulted in highly accurate prediction of hospitalization (92%). This tool should be tested in future studies with new subject data. Upon further development, we envision a simple mobile application to aid in the decision-making process for clinicians in areas with limited resources.
Read the full publication at PLoS Neglected Tropical Diseases.